Background: During transcatheter arterial chemoembolization (TACE), the delivery of a guidewire to the lesion site is a critical step, making the analysis and positioning of guidewire morphology crucial for both robotic systems and physicians in interventional surgeries. Current research on guidewires often faces challenges such as a low image signal-to-noise ratio and severe class imbalance. To overcome these issues and enhance the practical delivery of guidewires in clinical settings, this study introduces a comprehensive dataset for guidewire delivery during TACE and develops a specialized deep learning model for segmenting guidewire morphology in X-ray fluoroscopic images.
Methods: We retrospectively collected 2,839 X-ray images acquired under real-time guidance from 38 subjects and manually annotated the guidewires. We proposed a deep learning-based guidewire segmentation method, which integrated two effective modules designed in this study: a bilateral feature fusion (BGA) module and a lightweight gated attention (SDA) module, achieving precise segmentation of guidewires in intraoperative images.
Results: Quantitative and qualitative assessments were performed on 903 clinical images from 27 X-ray fluoroscopy videos. The segmentation network proposed in this paper demonstrated superior performance, achieving an area under the curve (AUC) of 91.64%, a Macro-F1 score of 85.63%, and a Dice coefficient of 71.29%.
Conclusions: This study introduces a novel guidewire segmentation method specifically designed for clinical TACE. It not only assists physicians during interventional procedures but is also expected to be integrated into the intelligent systems of vascular interventional surgical robots, enabling robotic assistance in the future interventional surgeries.
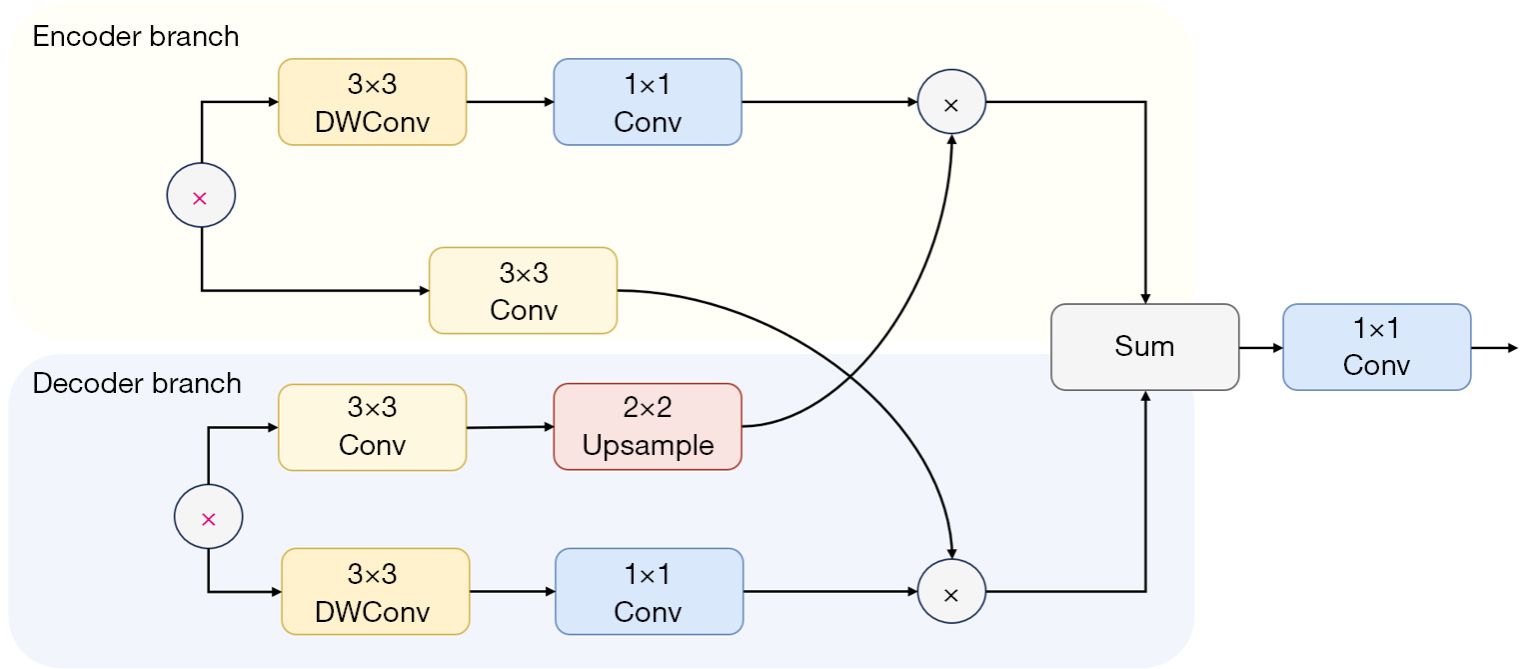
文章链接:https://qims.amegroups.org/article/view/136604/html